Soon we’ll all be in the Matrix, with agents doing our work. Hopefully, none of them will be like Agent Smith. Jokes aside, AI Agents are a growing focus in the GenAI ecosystem. Think of them as digital twins of employees, each designed to fulfil a specific role within an organization. Let’s break down the anatomy of AI Agents—and get into what makes them tick! And now about this…
AI Agents are rapidly evolving from abstract concepts into practical tools, with organizations increasingly deploying them to automate and support human work. However, whilst AI agents are hyped as transformative technology, some experts—like analysts at Gartner—warn that these systems are still in their early stages, with significant hurdles to overcome before they reach their full potential. Let’s look at some numbers: by 2025, 30% of enterprises will use AI-augmented testing strategies; by 2026, over 100 million workers will interact with AI “virtual colleagues”; and by 2027, half of all enterprises will implement responsible AI governance programs, with open-source AI usage increasing significantly.
So, what are the trends to support this? Four of them stand out: Autonomous Agents: The are are capable of executing complex tasks with minimal human intervention. Unlike traditional chatbots that need constant prompts, autonomous agents follow high-level instructions and autonomously manage sequences of actions. To reach their potential, AI models must improve in reasoning, memory, and contextual understanding. Multimodal Agents: Many models now handle multiple data types (text, code, images, video), expanding their application scope but also increasing complexity. Open-Source Agents: Demand for customization is growing, with businesses favouring open-source models for flexibility in deployment, whether in the cloud, on-premises, or at the edge. Edge Agents: Smaller models are being developed for constrained environments, like mobile devices, to provide acceptable performance without relying on the cloud.
Yet, despite the optimism, some organizations are experiencing the “slope of disillusionment” due to unmet expectations. And we’ve heard this so many times—especially from Gartner! Some of these challenges include high costs, as companies often underestimate expenses for data preparation, processing, and infrastructure. Then there’s the talent shortage, with intense competition for AI expertise as AI initiatives scale. Explainability and reliability issues, such as “hallucinations” and opaque decision-making, also limit adoption. Finally, software costs are rising as vendors embed AI agents into products and raise prices, adding to the cost barrier.
As these trends reshape the landscape, it’s clear that AI agents are inching closer to mimicking human roles in organizations. But to truly function as digital stand-ins for employees, they need a well-defined structure—a blueprint, if you will—that guides their actions and interactions. This involves more than just answering questions; it means embodying a specific persona, following nuanced instructions, and managing tasks with human-like reasoning. To support these capabilities, AI agents must be equipped with robust memory, allowing them to track context within a session, recall past interactions, and even delegate tasks to other specialized agents when needed. This structured approach helps AI agents navigate complex workflows, adapt in real time, and integrate seamlessly into business operations.
But that’s not all. Advanced agents need access to tools, like CRMs or APIs, to handle complex tasks that go beyond simple Q&A. Additionally, learning and adaptability allow agents to refine their responses over time, adjusting to user feedback or updated guidelines. And finally, there’s safety and bias mitigation, crucial elements to ensure responsible outputs by filtering harmful content and reducing biases. These components work together, creating AI agents that not only support but enhance human workflows.
Bottom line: The structure of AI agents mirrors human workflows, making them adaptable and effective in roles traditionally filled by humans, especially in customer support and related applications. So, let’s hope these agents will be less like Agent Smith and more like helpful teammates, ready to tackle the repetitive tasks while we focus on the bigger picture. See you on the next episode.

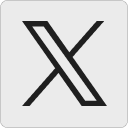



